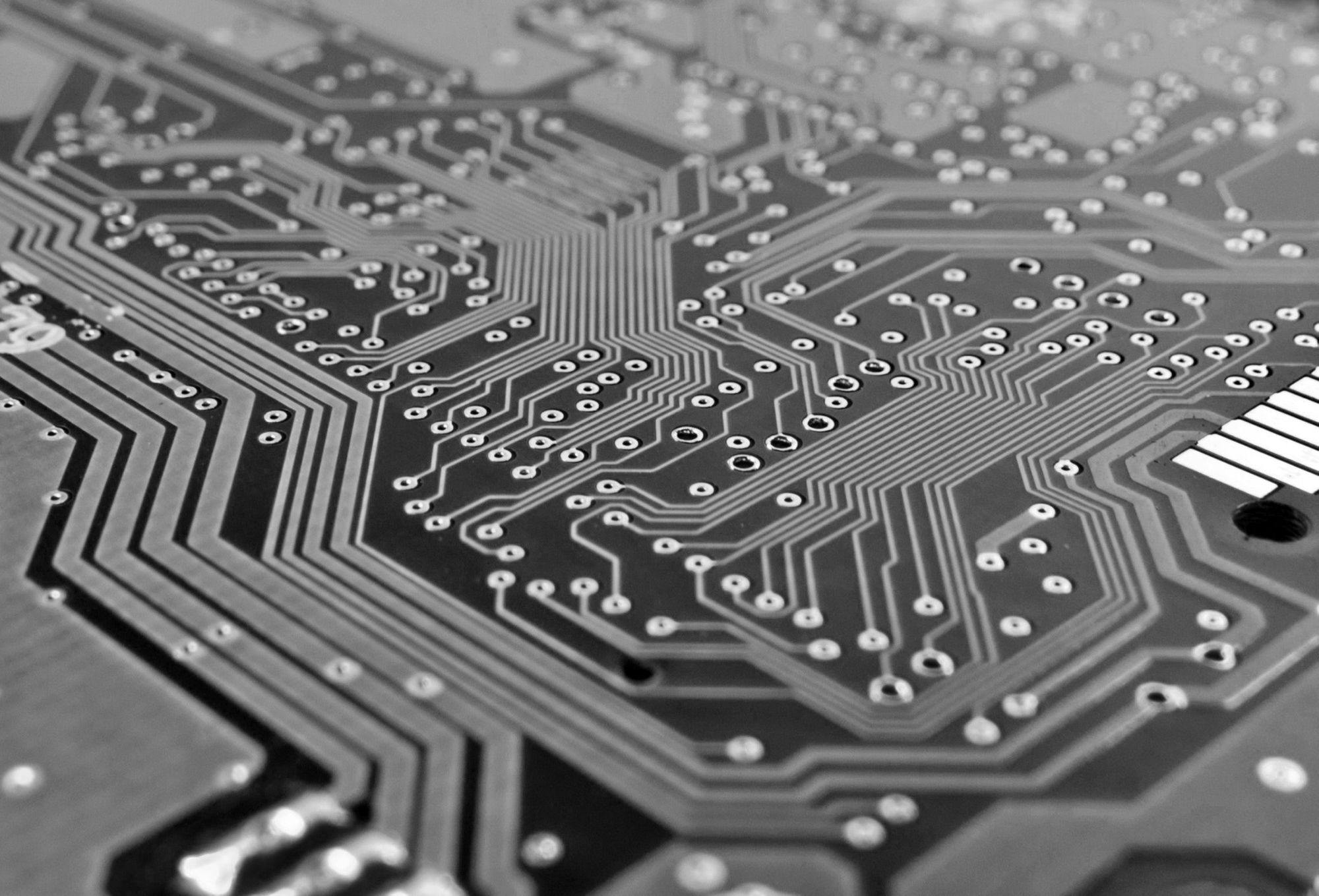
5 Best Practices for Facility Maintenance Planning in the Digital Era
In our interactions with clients, we find that many are mandated with digital transformation initiatives for their facilities, but are struggling with the transition because much of the needed information is locked in scanned documents that are tough to access. This impedes the implementation of intelligent preventive and predictive maintenance. Lagging competitors is not an option—especially with the havoc wreaked by coronavirus and the OPEC price wars in the last couple of months.
It’s to be expected. All of this technology emerged fairly recently, and it’s hard to figure out the best path forward. Here are the best practices we recommend for moving your facility maintenance planning strategy into the digital era:
- Choose a standard to adhere to.
The relevant standards are well known: ISO 16354/13250 for content classification, ISO 14224 to gain physical context, and ISO 55000 to establish operating context. The standards guide your team on effectively collecting and organizing the relevant data for your facilities. This empowers information access and exchange among all parties involved in the process, from manufacturers to facility owners.
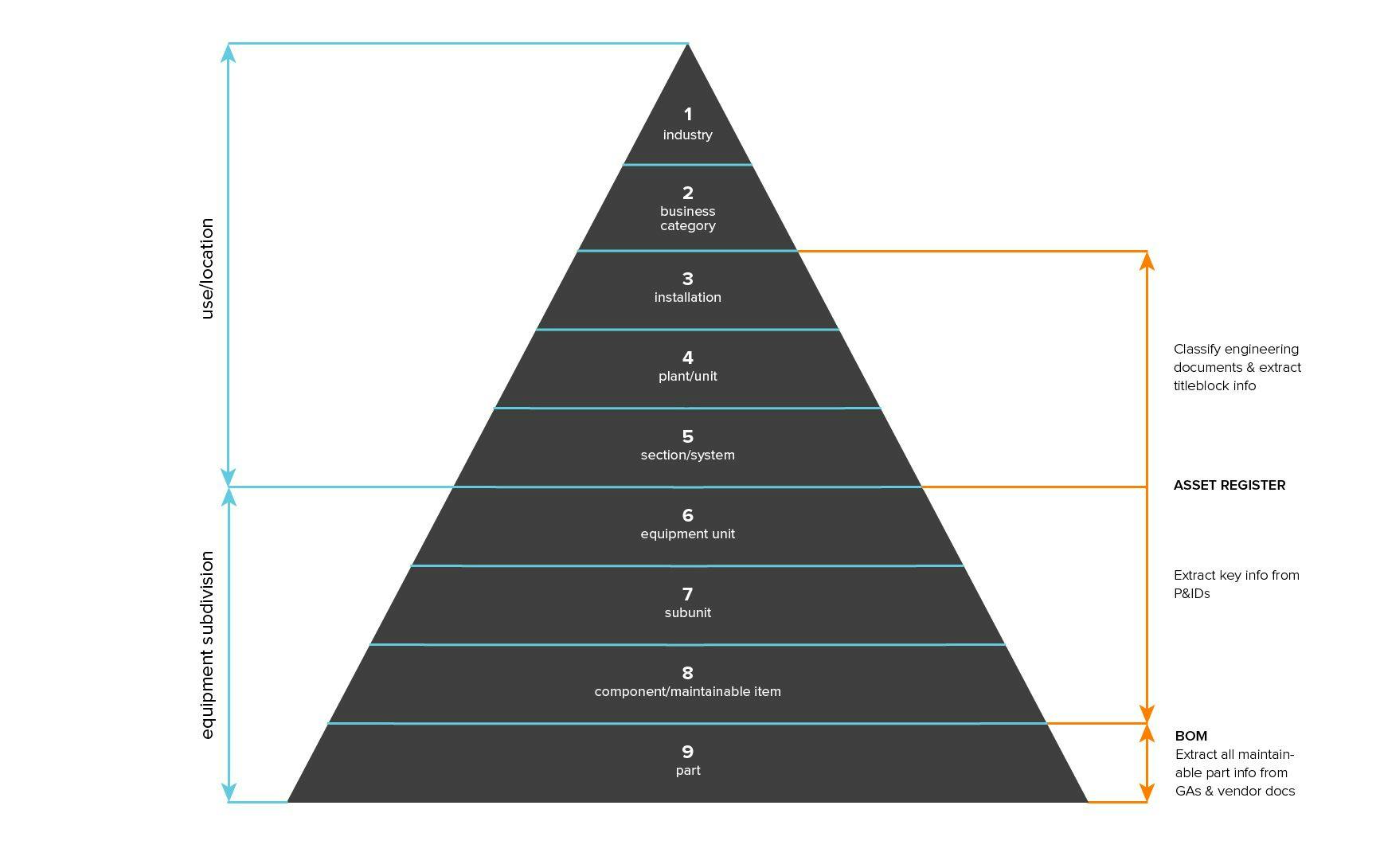
How to complete levels 3 to 9 of the ISO 14224:2016 standards
- Find the relevant data.
Most of the critical information is probably lost in convoluted file directories containing duplicate files, photos, and downright strange documents (we’ve seen some weird scans!). You need to find and process P&IDs, SLDs, SPIR tables and other mission critical data. - Use machine learning.
Depending on the number of facilities you’re dealing with, human-only effort can take over a decade of man years to find the relevant documents; extract information from title blocks and P&ID tags; and generate a comprehensive BOM for all your facilities. We’re not making that number up. Crazy, right? But through computer vision and natural language processing (NLP) techniques, machine learning can speed up that process by at least 40x. - Still, keep humans in the loop.
No false expectations or magic here—there’s no doubt that machines cannot achieve 100% accuracy. If someone claims otherwise, it’s because they gave it rules for a very small, very specific use case, or their algorithm is overfitting to the task. Regardless, machine learning will still get you 90% or more of the way, in a significantly shorter period of time. Just bring in a team of humans to review and remediate machine errors for data you need to be 100% correct. For a more in-depth breakdown on which steps should be tackled by machines and which ones require human intervention, check out our blog post, [Creating a Hierarchical Asset Register Using AI](https://cenozai.io/blog/creating-a-hierarchical-asset-register-using-ai/). - Link relevant information.
When there’s an outage and your maintenance crew accesses the relevant P&ID for the fix, they’d also want to be able to quickly get the linked data sheets, line lists, and loop diagrams. You’d do that by matching equipment, tag, and other key IDs across the various documents and saving the information to your database. Ideally, your system has hotspotting capability—meaning you can click on a tag in a P&ID, for example, and immediately see all the other documents relating to it, and vice versa.
By keeping these five key practices in mind, your facility maintenance and data science teams can work together to build the appropriate data pipeline and machine learning models to analyze your engineering documents and prepare for Industry 4.0. They can then dump the information into spreadsheet software, like Excel, for remediation before loading into your database. Alternatively, if time is a key factor or you simply prefer an out-of-the-box solution, use a system of intelligence (SOI). Cenozai’s AssetBot supports your team through levels 3 to 9 of the ISO 14224:2016 standard, all in a single interface that’s designed for seamless human and machine collaboration.
Recommended Posts
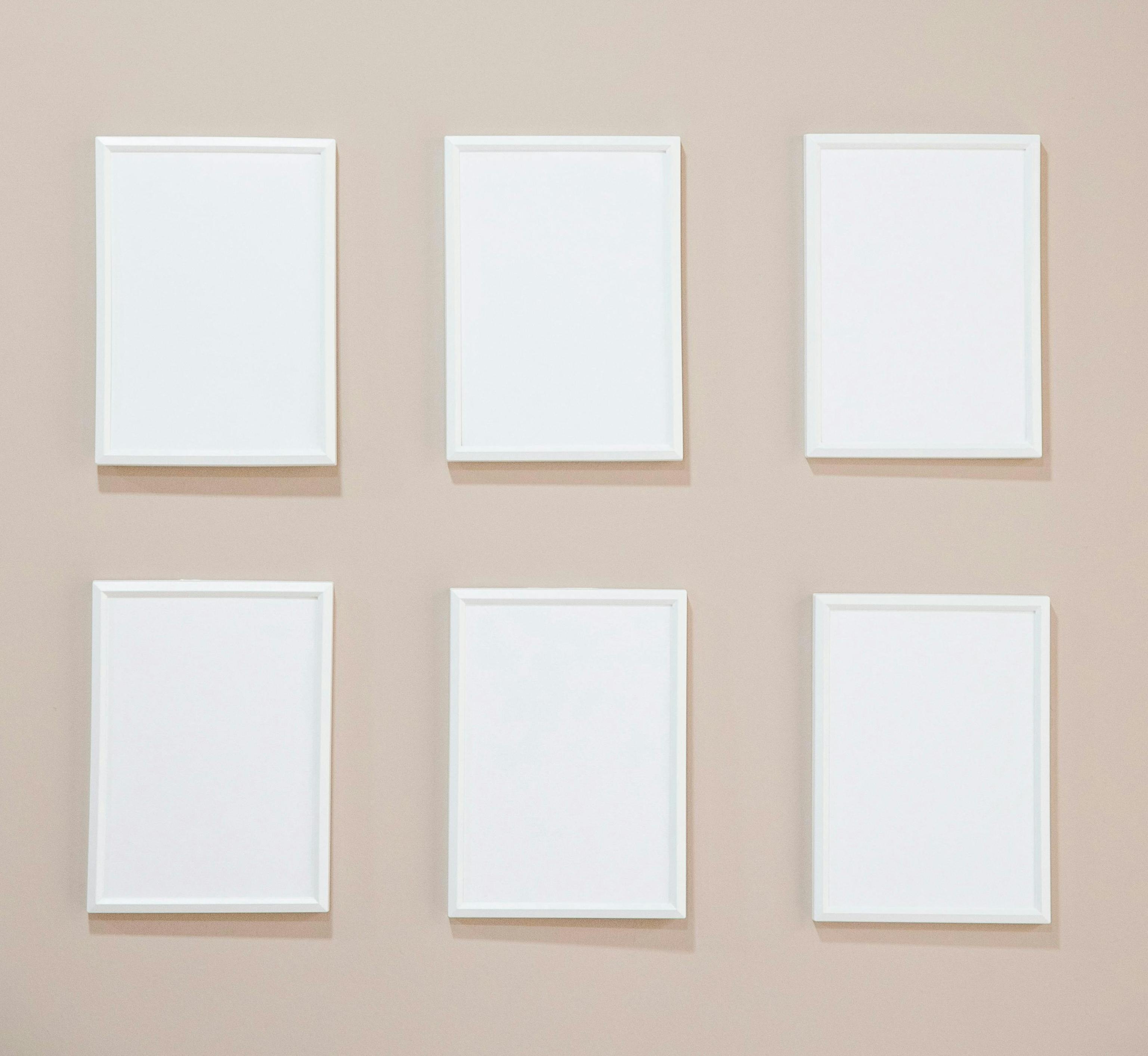
8/15/2024
A new era of enterprise search for facilities
(Part II): Data preparation
Before implementing new search technology, data cleanup and preparation is critical. Here's what you need to do.
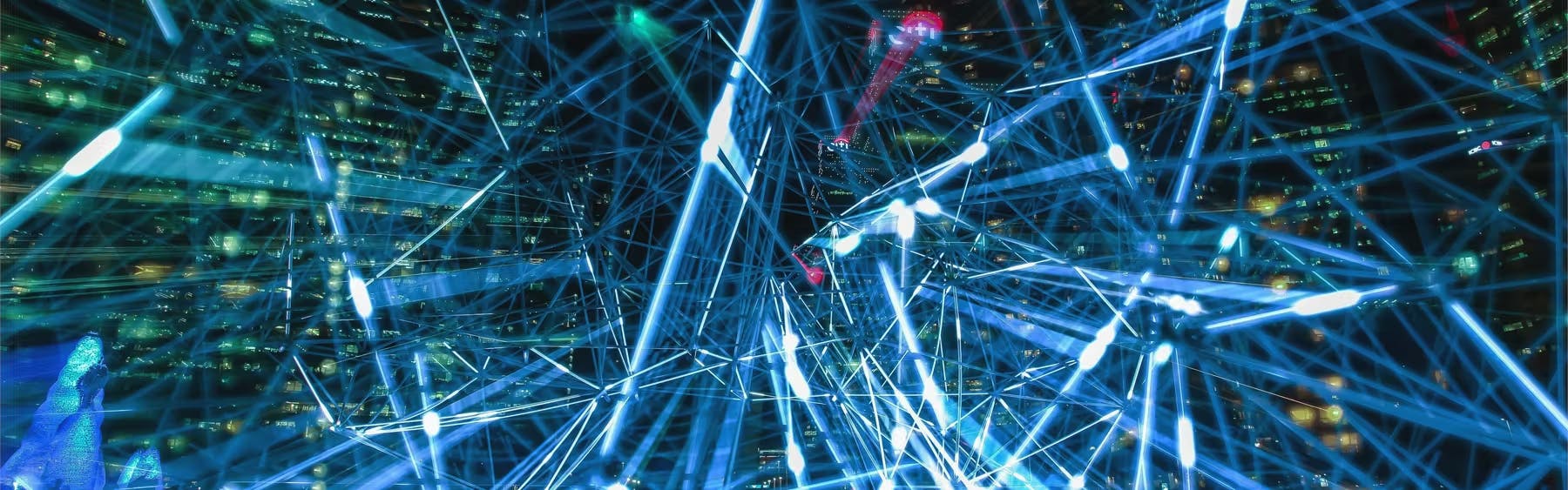
8/1/2024
A new era of enterprise search for facilities
Enterprise search is changing for facility operators—out with the file hierarchies and in with the information networks.
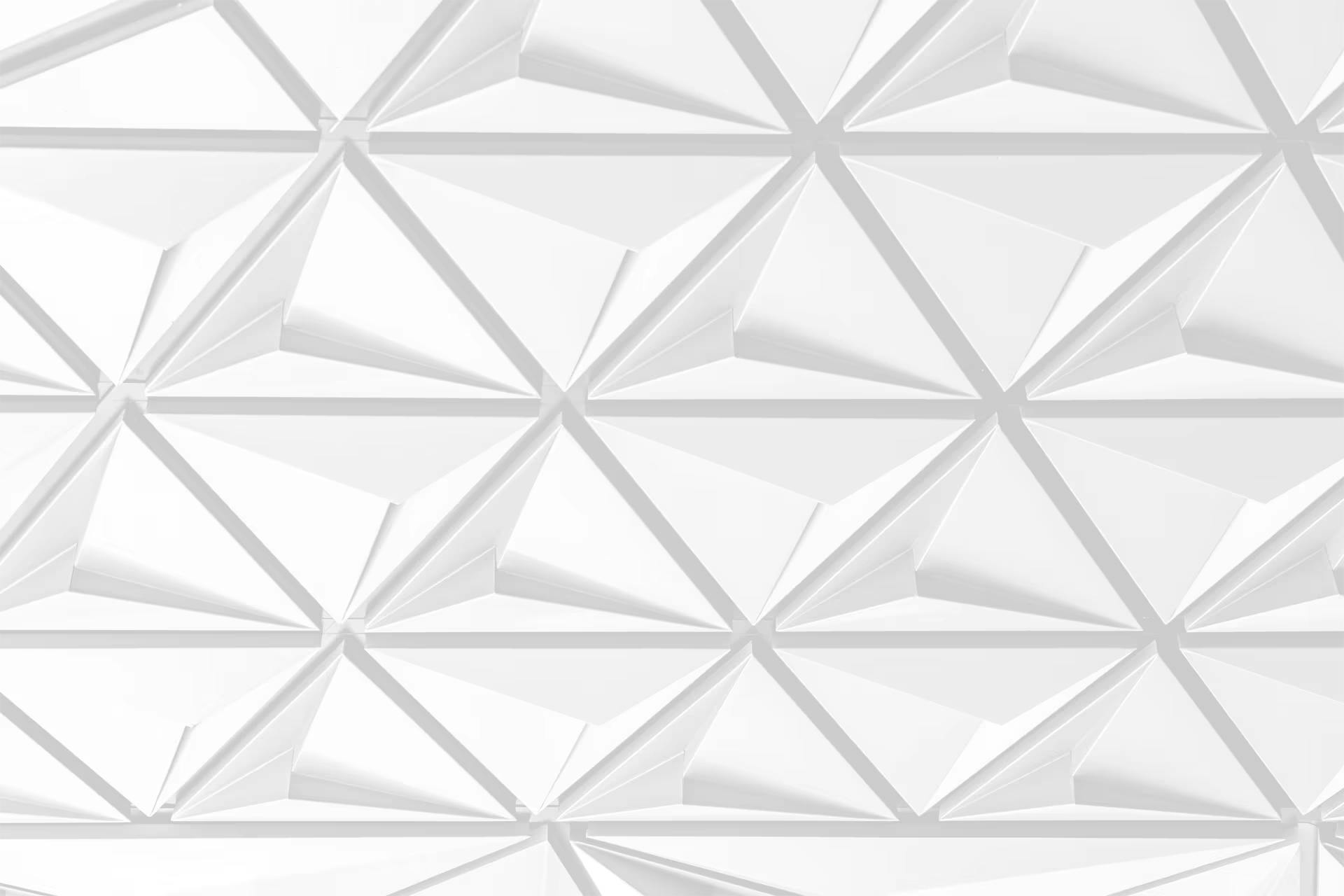
12/16/2022
Industry 4.0: The Unstructured Data Perspective
How to build a contextual platform that will be the launching pad for every one of your Industry 4.0 initiatives.